
The Assises of AI and Territories
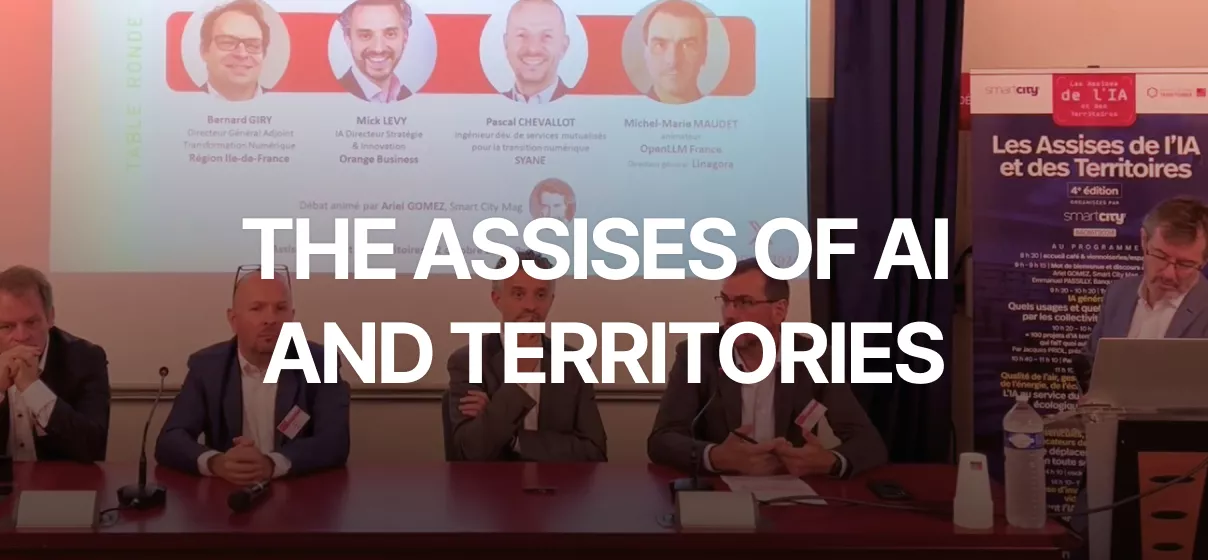
How can artificial intelligence (AI), including generative AI, support local and regional authorities and government departments in carrying out their public service missions? From mobility to security, via energy and the ecological transition (...), this day of the Assises de l'AI et des Territoires provided an opportunity to review a multitude of concrete applications of AI in our territories.
Generative AI is booming, generating as much enthusiasm as it does questions. Capable of producing text, images or even music from simple instructions, it is redefining the way we create and interact with technology. Its potential raises many challenges. From the reliability of the content generated to the protection of personal data, AI is forcing us to rethink our ethical, legal and economic frameworks.
The arrival of ChatGPT has profoundly transformed professional practices in many sectors, including public services. How can these generative AIs support local and national government employees in carrying out their missions? Michel-Marie Maudet, CEO and co-founder of LINAGORA, Bernanrd Giry, DG of Digital Transformation for the Ile-de-France Region, Pascal Chevallot, Development Engineer for shared services for the digital transition at SYANE and Mick Levy, IA Director of Strategy & Innovation at Orange Business, discussed this question at a round-table moderated by Ariel Gomez of Smart City Mag.
The challenges of generative intelligence
Michel-Marie Maudet identifies three major obstacles to the large-scale deployment of generative AI: the difficulty of demonstrating the added value for end-users, the high cost of the infrastructure needed to go to scale, and the complex integration of these technologies into the tools used on a daily basis, particularly when it comes to managing sensitive data.
" There are three obstacles to scaling up’ [...] It is difficult to objectify the perceived value for the end user [...] The cost of scaling up, since these infrastructures and services require investment [...] And the need to integrate these systems into the applications used on a daily basis. "
Mastering the handling and integration of sensitive or personal data in AI is a real added value:
" If we're dealing with HR issues, for example, we obviously can't do it with the technologies that are currently available to the general public. That's also what we're trying to offer, and it's this kind of use case that we're aiming for and that we think will bring more value to organisations, whether public or private. " Michel-Marie
Presentation of the OpenLLM community and LUCIE
The OpenLLM-France initiative, launched in June 2023, aims to promote diversity in the field of generative artificial intelligence (AI) through the development of 100% Open Source models. This approach aims to offer greater transparency, in particular by making training data public, thereby strengthening confidence in the uses of AI. It also makes it possible to pool efforts to reduce the environmental impact of training models.
LUCIE, the 1ᵉʳ Open Source generative AI model being developed by this community, will be free to use, with a balance of different European languages (French, English, Italian, Spanish, German) and technical documents (code, mathematics) to strengthen its reasoning capabilities. Unlike the large-scale models (LLM) of the American giants, Open LLM-France is focusing on ‘small-scale models’ (SLM), which are more sober and environmentally friendly, with the aim of making them accessible to everyone, even on simple infrastructures such as a conventional PC.
" The aim of this community is to develop diversity in the field of generative AI, with one objective: to train one or more 100% open source generative AI models. Michel-Marie Maudet
What resources do we have to deal with international companies?
The effort required to develop an Open Source generative AI model is quite considerable,’ confirms Michel-Marie. To train a model, you need (1) data, (2) computational resources and (3) talent.
For the LUCIE data, the community collected 3,000 billion words without using synthetic data or data from the Internet, in order to guarantee greater transparency and ethics, Michel-Marie adds:
" When you start to open the datasets (...), you realise that there are many, many things that you would not like to see appear. In addition, you should know that all the models I've worked on today certainly contain personal data that belongs to you or works protected by copyright. "
In terms of computational resources, access to the Jean Zay supercomputer has enabled the model to be trained free of charge, thanks to partnerships with public bodies. The project currently uses 512 H100 cards in parallel, consuming around 700,000 GPU hours for training.
Michel-Marie explains :
" 45,000 for a card is a lot of money! That's why we've created an open community. This kind of community is of interest to everyone: the scientific, academic and research worlds. So we very quickly got in touch with the people in charge of national computing resources, including an entity called GENCI, and you've no doubt heard of this famous Jean Zay machine."
Lastly, in terms of talent, France benefits from high-quality academic training in the field of AI, enabling it to mobilise a high-performance technical team with people from a variety of institutions:
" There are currently around thirty people training Lucie: ten from LINAGORA, and 20 others from research labs working with us (CEA, LORIA, IDRIS). One of the objectives of this community was to be able to broaden the field of expertise." Michel-Marie Maudet
LLM or SLM ?
In what situations would it make more sense to enrich the data in an LLM (Large Language Model) rather than use smaller models such as SLM (Small Language Model) or PLM (Personal Language Model)?
The strategy of specialised models, adopted in particular by OpenAI with its latest models, is booming in the AI industry. These smaller, customised models are recommended for customers, along with model hubs that allow several approaches to be tested for different use cases. However, training these models still presents many challenges, particularly related to data: user rights, biases, and gendered representation.
Michel-Marie :
" Typically, all of today's models use data from Wikipedia, which can be considered a reliable source, but overall, 90% of Wikipedia's content is either constructed or validated by men. So what do we do? How do we treat our data? "
These are unavoidable biases or ‘preferences’, often linked to data filtering choices. This is why Michel-Marie advises limiting the fine-tuning of models, as this is costly and complex to maintain. Solutions such as RAG (retrieval-augmented generation) are recommended for current use cases in local authorities. In addition, it should be emphasised that many tasks can still be carried out using symbolic AI or simple algorithms, without the need for sophisticated generative AI models.
How do you capture collective intelligence?
The first interesting use case: capitalising on collective intelligence during meetings. The aim is to develop tools capable of automatically recording, transcribing and summarising meetings, while enabling intelligent interaction with this content. A tool with multimodality, in other words, capable of interacting with content generated by voice rather than by keyboard, thus facilitating access to specific information, such as summaries of previous interventions.
On this subject, LINAGORA's Managing Director describes a number of concrete projects, such as theequipment of meeting rooms for the European Commission, and the disconnected and secure solutions developed for the French Ministry of the Armed Forces, which make it possible to record and interact with the summaries of sensitive meetings in secure environments. The idea is to better capitalise on collective intelligence and make it accessible in an efficient way over time.